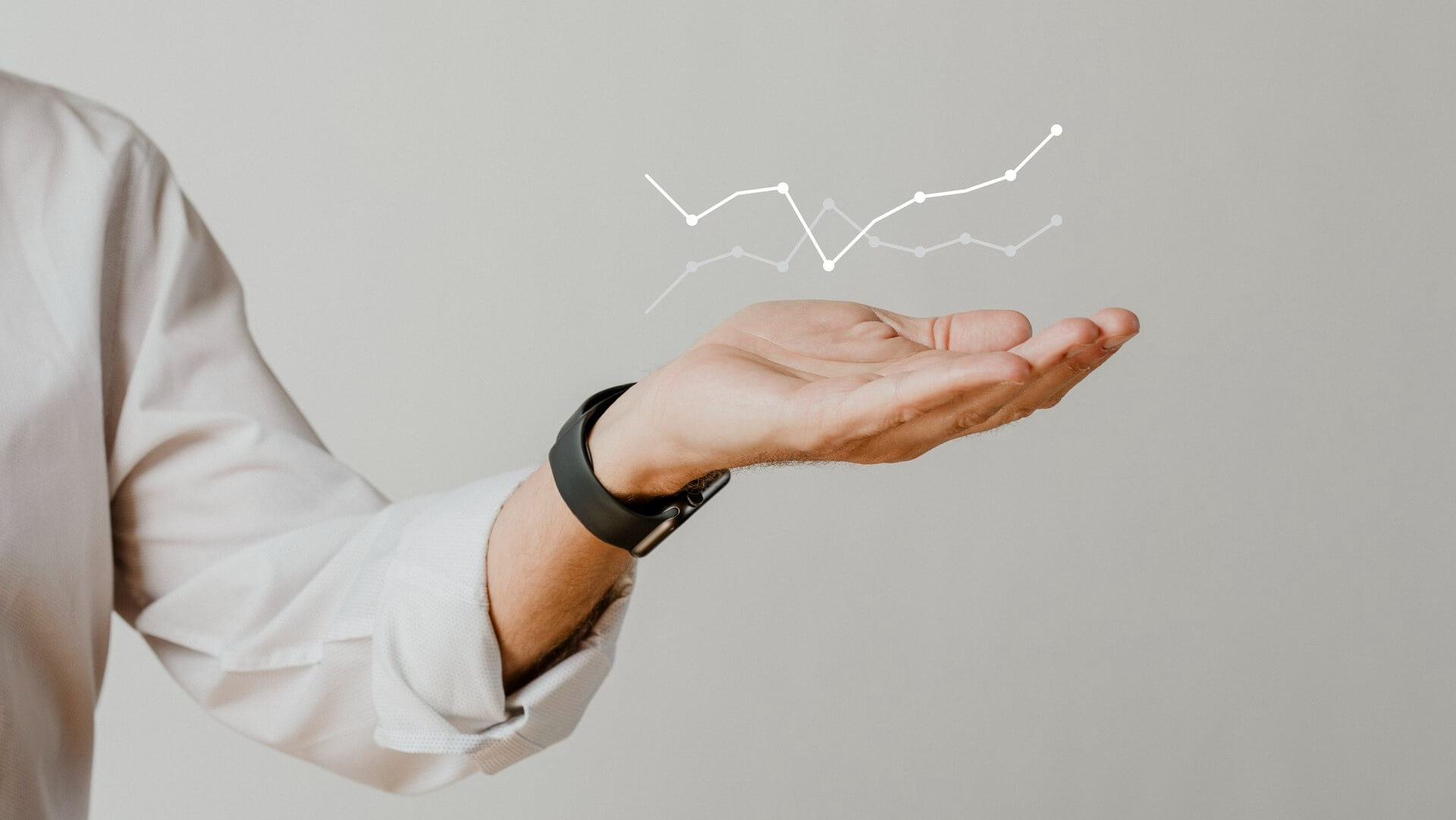
The future development of big data has three main directions: big data platform cloud native biology; lake warehouse integrated; big data and artificial intelligence to reshape the value of data, we will interpret the three directions one by one.
Big data platform cloud native biology is an inevitable trend
Big data system is a high complexity system, the traditional big data system operation and maintenance costs are very high, however, most of the enterprises today are facing the growing amount of data, various types of data in real time and intelligent processing needs, enterprises urgently need to reduce the cost of operation and maintenance, and hope to produce through the data mining to support the business side of the insight and prediction!
As a result, cloud-native big data platforms are welcomed by enterprises because of their highly elastic scalability, multi-tenant resource management, massive storage, heterogeneous data type processing and low-cost computational analysis, which is the inevitable development trend of big data systems.
Running big data on the cloud and providing it to users in the form of cloud services can greatly enhance the serviceability of enterprises, and users can directly perform value mining on the cloud. Moreover, when vendors provide big data technology through cloud services, many new capabilities become transparent, and enterprises can seamlessly provide their own services to users without having to go through fumbling and integration.
In order for enterprises to be able to run their business better on top of the architecture of the cloud, they are currently generally using architectural layer solutions. Cloud-native supercomputing, which incorporates the powerful arithmetic of high-performance computing (HPC) and the security and ease of use of cloud services, seems to be the best effective solution at present. But the fact is that the software layer upgrade is still more or less affected by the hardware layer. So, why not change the direction and think about how to use hardware capabilities to improve data processing efficiency.
The "Lake Warehouse" is an emerging architecture to solve the problem of real-time data
With the rise of artificial intelligence and other technologies, the scale of data is getting bigger and bigger, and the types of data stored are getting richer and richer. Compared with text, the demand for storage of pictures, sound and video with larger volume explodes. In the face of these massive data governance needs, data warehouses and data lake architectures are widely used by enterprises.
Many currently believe that data warehouses that are domain-themed, integrated, stable, and able to reflect historical data changes are no longer able to meet the data needs of artificial intelligence and machine learning technologies and are beginning to gradually go downhill, and data governance architectures are gradually crossing over from data warehouses to data lakes.
In fact, most enterprises currently have at least one or more data warehouses serving various downstream applications, and putting all the raw data into the data lake may enhance the difficulty of using data, which is not a small challenge for enterprise data governance; in addition, from the aspect of real-time, the data lake can't do real real-time.
However, the use scenario of enterprise data has changed dramatically, and the demand has shifted from offline scenario to real-time data analysis scenario. After the development of data scale to a certain extent, the shortcomings of offline data will be more and more prominent, enterprises have higher requirements for real-time data governance, hoping that the data obtained from the business side can be immediately cleaned and processed, so as to meet the data-based mining, prediction and analysis.
Therefore, as an emerging architecture, "Lake Warehouse All-in-One" combines the advantages of data warehouse and data lake, and achieves similar data structure and data management functions as data warehouse on the low-cost storage similar to data lake, and shows unique advantages in scalability, transaction and flexibility, which is a better solution to the current enterprise data governance needs. It is a better solution to address the current needs of enterprise data governance.
"The integration of AI and big data" reshapes the value of data
Data shows that more than 85% of AI projects end up in failure and are not really delivered. The reason for this is that the AI models and algorithms being run in the lab are not the same as what is required to actually get to the production environment or business scenario.
Thinking back, when building some AI architectures, the common practice is to use a big data processing platform, then process the data, and then copy the data to another AI cluster or a deep learning cluster for training. Obviously, the process of data copying will incur certain time costs and transplantation costs, solving this problem can greatly improve the efficiency of enterprise research and development, and quickly achieve cost reduction and efficiency.
In order to support the processing of big data, the first thing Intel does in "AI+ Big Data" is to build a unified big data AI platform and cluster - Intel BigDL, which is a distributed deep learning library for Spark and can run directly on top of existing Spark or Apache Hadoop clusters, and can write deep learning applications as Scala or Python programs.
MasterCard's enterprise data warehouse is built on top of a distributed big data platform that uses Intel BigDL to build AI applications directly, unifying big data processing with artificial intelligence processing and helping the platform support more than 2 billion users.
The hundreds of billions of transaction data on the platform have trained a very large number of AI models, the largest of which is running on more than 500 Intel servers for large-scale distributed training in a single task, and a large-scale AI model is trained within almost 5 hours to improve various AI capabilities and realize the support of super large-scale user volume.